Predictive analytics holds incredible promise, but for many businesses, the path to results is filled with unexpected hurdles. Teams often start off excited about the potential, only to run into predictive analytics challenges far sooner than expected like messy data, mismatched models, and unclear success metrics.
The result? Confusion, delays, and stalled momentum before any real value is realized.
As Eric Schmidt, former CEO of Google, once said, “The biggest risk is not taking any risk.”
But the second biggest? Taking the wrong one.
That’s when the doubt sets in. Should we keep going? Did we choose the right tools? Is our data even usable?
While teams are figuring the answers out, the market keeps growing. And that adds pressure. Just like the predictive analytics industry is projected to grow from $11.5 billion in 2023 to $61.9 billion by 2032, with a 21.2% CAGR, according to Market US.
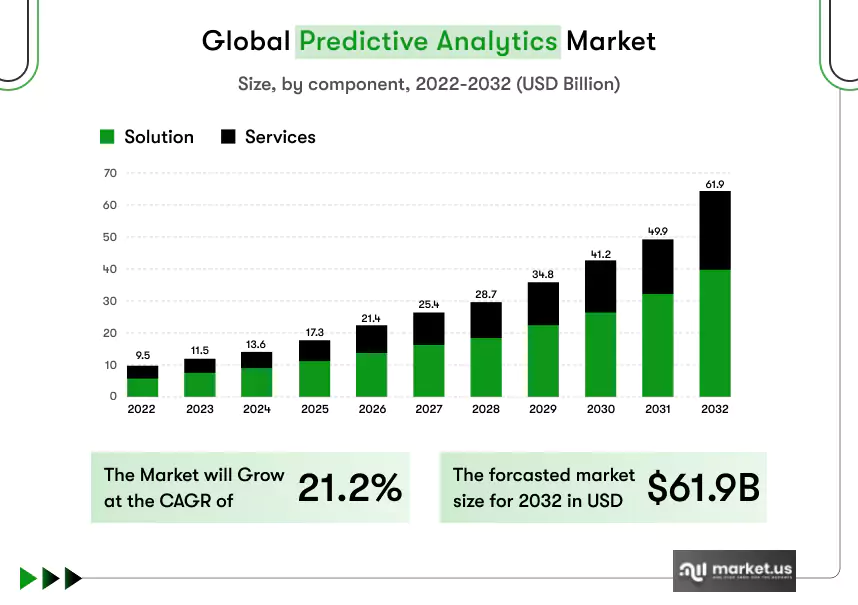
This kind of growth shows the urgency. But without a clear plan, many companies fall behind.
This guide will help you avoid those mistakes and tackle real challenges of predictive analytics with confidence.
Not sure what predictive analytics really means for your business?
Start with this quick predictive analytics guide to get the basics. Then come back here, we’ll show you how to avoid the mistakes most teams still make.
Why Do Predictive Analytics Projects Fail in Businesses?
We’ve worked with companies across industries such as retail, healthcare, logistics, and finance who’ve all faced the same story.
They get excited about predictive analytics. They invest in tools, hire data talent, and launch with confidence. But within months, the project slows down. The insights don’t reach the right people. The model sits unused. The excitement turns into confusion.
And it’s almost never because the model was wrong. It’s because small missteps early on created bigger problems later. This is where most predictive analytics challenges begin, quietly, but deeply.
Let’s break them down.
Teams start with tools, not business goals.
Most companies choose platforms and models before defining what they actually want to predict. Without a clear question, the project lacks purpose. Even the best model won’t matter if it doesn’t solve the right problem. This is one of the earliest challenges of predictive analytics, and it leads to misaligned outcomes and wasted budget.
Data looks available, but it’s not usable.
The data might be there, but it’s often outdated, messy, or spread across disconnected systems. Predictive analytics relies on clean, structured, and relevant data. If that’s missing, your insights will be too. This is why most early predictive analytics challenges and solutions start with a deep data clean-up.
Business teams and data teams don’t speak the same language.
Leaders talk about revenue, growth, and retention. Data teams talk about features, training sets, and model accuracy. When those conversations don’t align, confusion follows. This misalignment is one of the biggest challenges faced by people in predictive analytics, and it leads to missed expectations and stalled momentum.
The model works, but no one uses it.
You built it. It runs. It performs. But the people who need it don’t trust it, or worse, don’t even know how to use it. A lack of adoption turns powerful predictions into shelfware. This is one of the most frustrating predictive analytics challenges, because technically, everything works, but no one acts on it.
There’s no plan for what comes next.
Predictive analytics isn’t a one-time job. Models need updates, feedback, and long-term integration into your systems. Without a post-launch plan, the model quickly becomes outdated. This slow fade-out is where long-term challenges of predictive analytics begin, often quietly, but at a high cost.
All of this is happening while expectations around analytics keep growing. With the rise of AI & machine learning in predictive analytics, teams feel even more pressure to show fast results. But without the right foundation, the advanced tools don’t matter.
Every one of these problems is avoidable. But only if you recognize them early. That’s what separates successful projects from the ones that fail quietly.
In the next section, we’ll show how these challenges appear inside real business projects, and what to do about them.
Predictive Analytics Challenges That Can Derail Your Project
Predictive analytics opens the door to smarter decisions, faster insights, and real business value. But getting there isn’t always simple.
We’ve worked with teams that started strong, only to hit roadblocks they didn’t see coming. These are the predictive analytics challenges that often go unnoticed until the project slows down or fails to deliver results.
Businesses often turn to predictive analytics consulting at this point, hoping to get things back on track. But most of these issues are avoidable with the right approach from the start.
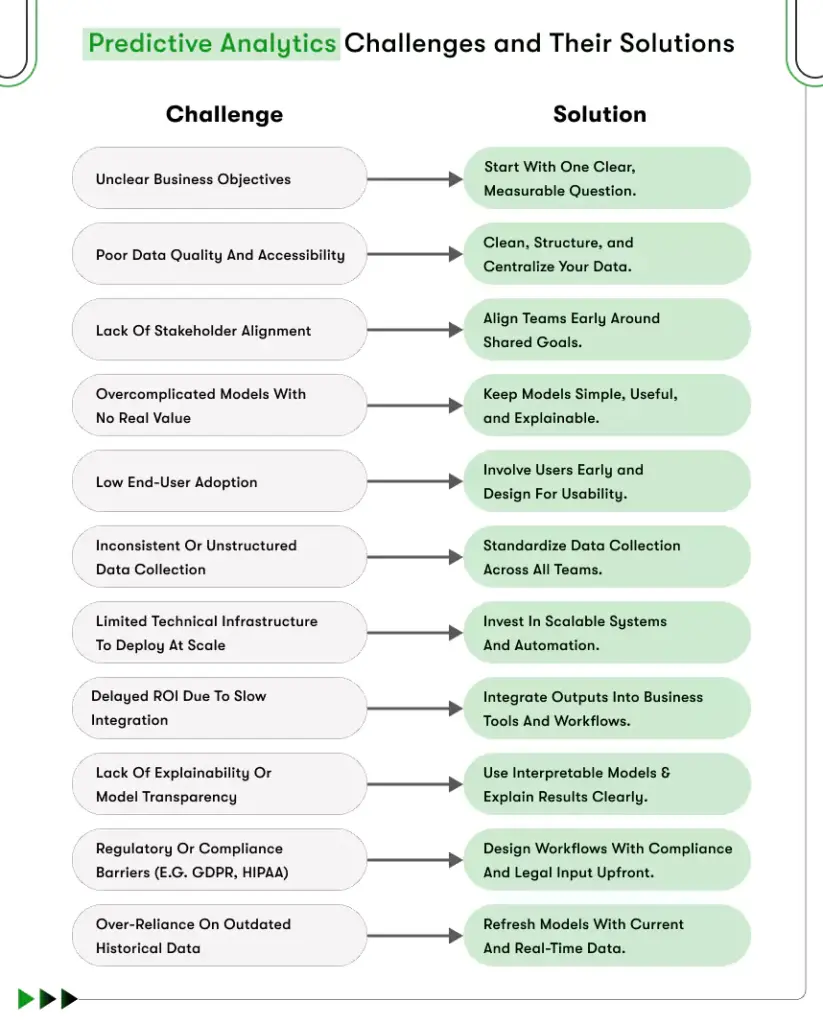
Challenge 1: Unclear Business Objectives
Many predictive analytics projects fall apart because no one defines the actual business problem. Teams jump straight into tools and data without knowing what they’re trying to solve. This leads to models that technically work but don’t support meaningful decisions.
Without a clear business objective, even the best predictions go unused. It’s one of the most common predictive analytics challenges we see in real-world projects.
The Solution to Avoid Unclear Business Objectives
Start every project with one specific, measurable business question. Skip vague goals like “reduce churn” or “improve efficiency.” Instead, ask questions like “Which customers are likely to cancel in the next 30 days?” or “How can we reduce delivery delays by 10% this quarter?”.
A sharp, focused question aligns your team, informs the model, and sets clear success criteria. If you’re unsure how predictive analytics works, don’t start with the tech. Start with the decision you want to improve.
Real-World Example
Slack offers a great example of this in action. Instead of focusing on general user growth, they identified one clear metric: users who send at least 2,000 messages are more likely to stick.
That became their success target. It shaped how they built their onboarding and product features. Because they had a specific goal from the beginning, their predictive models delivered insights that actually supported growth.
Challenge 2: Poor Data Quality and Accessibility
Most teams underestimate how messy their data really is. On the surface, there’s plenty of it, sales records, user activity, support logs. But once the predictive analytics process starts, they quickly realize the data is outdated, incomplete, or stored in disconnected systems.
This slows down the project and produces weak results. Even worse, teams often spend more time fixing data than building the model.
Poor input leads to poor output, and this is one of the most common predictive analytics challenges we see across industries.
The Solution to Avoid Poor Quality and Accessibility
The model is only as good as the data it’s built on. That’s why data readiness has to come first. Before any modeling begins, teams need to run a proper audit.
Clean the data, remove duplicates, structure it, and make it accessible to everyone who needs it. It’s not just about quality, it’s about usability.
Many predictive analytics challenges and solutions start with fixing the data problem, not the algorithm. Investing in a strong data foundation sets up every step after success.
Real-World Example
Netflix is a strong case where data quality came first. During the Netflix Prize competition, teams struggled with gaps and inconsistencies in the dataset Netflix provided. These issues made accurate predictions difficult.
Later, Netflix shared how their teams built data pipelines to clean and centralize viewing behavior, device usage, and account data before any modeling. That focus on clean input became a foundation for their highly accurate recommendation engine.
Challenge 3: Lack of Stakeholder Alignment
Predictive analytics projects often struggle because the people involved don’t share the same priorities. Business leaders focus on outcomes.
Data teams focus on models. Product teams think in terms of features or user behavior. When these groups don’t align early, projects move in different directions.
Miscommunication delays progress, creates confusion, and hurts adoption. This disconnect is one of the most overlooked predictive analytics challenges, and it slows down even the most promising initiatives.
The Solution to Avoid Lack of Stakeholder Alignment
Everyone involved in the project must understand the business goal and their role in achieving it. Start with a shared outcome, not just technical requirements. Align stakeholders from day one, business, data, product, and even operations.
Set expectations clearly: what decisions will the model support, and who will use it? This cross-functional clarity avoids duplicated effort, missed insights, and last-minute breakdowns.
Many challenges of predictive analytics can be avoided just by getting everyone on the same page early.
Real-World Example
Airbnb faced this during the development of their dynamic pricing tool. At first, engineers and data scientists focused only on prediction accuracy. But product managers and hosts cared more about usability and trust.
Once they aligned around the shared goal, helping hosts price smarter while feeling in control, the tool gained traction.
Airbnb avoided one of the most common challenges in predictive analytics by aligning their model goals with real user needs.
Challenge 4: Over Complicated Models with No Real Value
Some teams believe that the more complex or advanced the model, the better the results will be. So, instead of building something simple and useful, they focus on adding as much complexity as possible, extra variables, layers, algorithms, just to make the model seem impressive.
But in doing that, they often forget the actual goal: to solve a real business problem in a way that people can understand and use.
The Solution to Avoid Over Complicated Models with No Real Value
A simpler model that answers a real question is always better than a complex one nobody understands. Start by building a baseline model that’s easy to explain.
Validate it with users before adding layers of complexity. Involve both technical and business teams in testing and interpretation. Focus on clarity, not novelty.
Most challenges of predictive analytics at this stage aren’t technical, they’re about usability, trust, and communication between the model and the people it’s meant to help.
Real-World Example
Booking.com is known for testing and validating everything. When developing their pricing and conversion models, they started with simple, explainable models first.
Even though their team had the ability to build far more advanced systems, they focused on models that product teams and business managers could actually use.
This approach helped them avoid one of the key predictive analytics challenges and solutions, balancing complexity with clarity, without compromising business value.
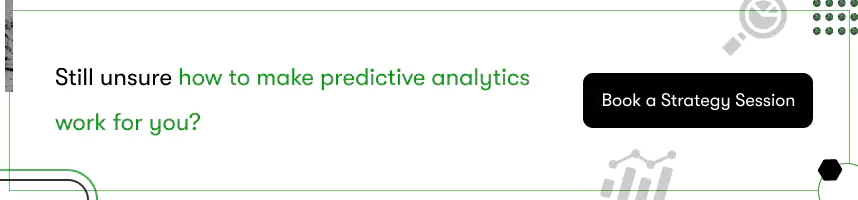
Challenge 5: Low End-User Adoption
A predictive model can be accurate, fast, and technically perfect, but if no one uses it, it’s a wasted effort. This happens more often than most teams expect. The insights are there, but users either don’t trust them or don’t understand how to apply them.
Sometimes the model feels disconnected from real decisions. Other times, teams were never included in the process.
As a result, adoption stays low. Among the most frustrating predictive analytics challenges, this one feels like failure, even when everything works behind the scenes.
The Solution to Avoid Low End-User Adoption
For any model to create impact, it has to be built with its users in mind. That means bringing them in early, asking how they make decisions today, and shaping the output around that process. If the prediction doesn’t translate into clear action, it won’t be used.
Teams also need context, training, and support, not just numbers. Many predictive analytics use cases fail simply because they overlook the human side. Adoption isn’t a phase. It’s part of the design from day one.
Real-World Example
LinkedIn faced this challenge with their “People You May Know” feature. The early model worked well, but user engagement stayed flat. The team realized that even though predictions were accurate, they weren’t relatable.
Once they changed how suggestions were displayed, adding mutual connections and user-friendly visuals,engagement jumped.
LinkedIn overcame a classic barrier by shifting focus from model output to user experience.
Challenge 6: Inconsistent or Unstructured Data Collection
Predictive analytics needs clean, consistent data, but most teams collect it in disconnected, unstructured ways. Different formats, missing fields, or siloed systems make the data messy before modeling even starts.
When it’s finally pulled together, it’s often too incomplete or chaotic to support useful insights. This is one of the most critical predictive analytics challenges because without structured data, even the best models fail.
The Solution to Avoid Inconsistent or Unstructured Data Collection
Start by standardizing how data is collected across teams. Define what’s needed, create shared formats, and train people to follow them. Don’t just collect more data, collect the right data, consistently.
Clean input sets the foundation for strong predictions later. Fixing this early avoids major slowdowns down the line.
Real-World Example
Spotify ran into this when building its recommendation engine. Data came from multiple services with no consistent structure.
Once they aligned how user behavior was logged, the model finally had what it needed. That shift laid the groundwork for features like Discover Weekly and personalized playlists.
Challenge 7: Limited Technical Infrastructure to Deploy at Scale
Many predictive analytics projects work well during testing but start breaking when it’s time to go live. The technical infrastructure simply isn’t built for scale. Some systems can’t process large data volumes.
Others lack automation or real-time prediction capabilities. In many cases, models sit idle because they can’t connect with production systems.
This is one of the more frustrating predictive analytics challenges, not because the model fails, but because the system around it can’t support success.
The Solution to Avoid Limited Technical Infrastructure to Deploy at Scale
Scaling predictive analytics requires more than just smart models. It needs strong infrastructure, fast data pipelines, and deployment-ready environments. Before launching, evaluate whether your stack supports version control, data refresh, model retraining, and integration.
Cloud services like AWS, Azure, or GCP offer flexibility, but need proper planning. When companies compare predictive analytics vs. traditional analytics, this is often the gap, they realize that predictive models demand far more agility and backend support.
Invest early in tools and workflows that make deployment seamless. It ensures the model doesn’t stay in a dashboard, it becomes part of real business operations.
Real-World Example Who tackled Limited Infrastructure Issue.
Uber ran into this challenge as it grew predictive systems for surge pricing, ETAs, and driver demand. Early models were siloed, with no easy way to scale or deploy.
To fix this, Uber built Michelangelo, an internal machine learning platform. It allowed teams to automate model training, testing, and deployment across products.
That move helped scale predictive analytics globally and made real-time predictions part of the everyday customer experience.
Challenge 8: Delayed ROI Due to Slow Integration with Business Systems
Many predictive analytics projects deliver solid predictions but still don’t show results on the ground. Often, the issue isn’t the model, it’s that insights aren’t plugged into business tools.
Teams work in silos, using disconnected platforms, so predictions don’t reach decision points. The sales team stays in CRMs, marketing sticks to email tools, and finance never sees real-time inputs.
This disconnect is one of the most expensive predictive analytics challenges because it slows down value, even when everything technically works.
The Solution to Avoid Delayed ROI Due to Slow Integration with Business System
To generate impact, predictions must flow into the systems people already use. That includes CRMs, inventory platforms, marketing tools, and even financial planning dashboards. Set up automation to deliver insights where actions happen, not in separate dashboards.
Companies that integrate early avoid stalled momentum and delayed returns. The ROI of Predictive analytics increases dramatically when output drives decisions without extra steps or manual transfers.
Many of the most common challenges of predictive analytics show up at this stage, not because the model is wrong, but because it’s disconnected from action.
Real-World Example
Target faced this challenge with its promotion and inventory prediction models. While the forecasts were accurate, store teams didn’t feel the impact because systems weren’t connected.
Once target integrated model outputs into supply chain and in-store planning tools, results followed quickly.
Teams aligned faster, shelves were better stocked, and marketing efforts matched demand. Integration turned accurate predictions into measurable operational wins.
Challenge 9: Lack of Explainability or Model Transparency
Even a highly accurate predictive model can fall short if no one understands how it works. Business leaders question the results. Regulators demand clarity. End users hesitate to trust something they can’t explain.
When a model feels like a black box, it creates friction, resistance, and legal risk. This is one of the most sensitive predictive analytics challenges, especially in high-stakes environments where decisions impact customers, revenue, or compliance.
The Solution to Avoid Lack of Explainability
Build transparency into the process from the start. Use interpretable models when possible, and when advanced models are needed, pair them with clear summaries or visualizations.
Techniques like SHAP values, LIME, and feature importance scoring can help translate complex outputs into plain language. Involve non-technical stakeholders in reviewing model logic before launch.
Transparency doesn’t just build trust, it’s often required. Many predictive analytics challenges and solutions begin not with code, but with communication between data teams and the rest of the business.
Real-World Example
Bank of America encountered this when building credit risk prediction models. Early versions were accurate but not easily explainable to auditors or underwriters. The data science team simplified model logic and added explainability layers that broke down key drivers behind each prediction.
This allowed compliance teams to approve the system and enabled staff to use the model confidently in real decisions. It’s a real win in overcoming challenges faced by people in predictive analytics, where trust is just as important as accuracy.
Challenge 10: Regulatory or Compliance Barriers (e.g. GDPR, HIPAA)
Even the most advanced predictive analytics systems can get blocked by regulations. Laws like GDPR, HIPAA, and CCPA define how data is collected, stored, and used. If your model processes personal or sensitive data, you’re dealing with legal boundaries that can’t be ignored.
Many companies hesitate to deploy because they’re unsure if the system complies. This makes regulatory constraints one of the most serious predictive analytics challenges, especially for teams working with health, financial, or behavioral data.
The Solution to Avoid Regulatory or Compliance Barriers (e.g. GDPR, HIPAA)
Compliance needs to be built into your predictive analytics workflow from day one. Work with legal and data governance teams before collecting or processing data.
Make sure consent is recorded, data sources are audited, and usage aligns with local laws. Use techniques like data anonymization, aggregation, or federated learning when dealing with personal information.
Predictive models that are compliant by design avoid slowdowns, fines, and reputational damage. Many challenges of predictive analytics tied to compliance are not technical, they’re the result of leaving legal teams out of early conversations.
Real-World Example
A major European health-tech company developing predictive diagnostics hit a wall with GDPR. Their model used patient-level data without full user consent for secondary analysis.
Before they could launch, they restructured the pipeline to anonymize identifiers and only process aggregated insights. That move satisfied regulators and let the model go live safely.
It’s a clear example of how ignoring early legal input creates one of the most damaging challenges faced by people in predictive analytics, regulatory delays after months of work.
Challenge 11: Over-Reliance on Historical Data That No Longer Reflects Reality
A lot of teams trust historical data too much. They build models that reflect how things worked two years ago, not today. But customer behavior, supply chains, and markets change fast.
The model may be accurate on paper, but completely off in real life. This is one of the most overlooked predictive analytics challenges because it doesn’t break things, it just quietly leads you in the wrong direction.
The Solution to Avoid Over-Reliance on Historical Data That No Longer Reflects Reality
Models need fresh data. That means checking if your historical inputs still reflect what’s happening now. Add signals like market shifts, seasonality, or external data when needed. Set up pipelines to refresh models regularly, so they stay relevant.
As predictive analytics trends shift toward real-time insights, outdated data becomes a silent threat. Many predictive analytics challenges and solutions can be avoided just by asking, “Is this data still true?”.
Real-World Example
During COVID, Amazon’s older demand forecasts broke down fast. Consumer behavior changed overnight. Instead of sticking with outdated inputs, they retrained their models on current data, shopping trends, delivery times, and user behavior.
That one move helped them stay accurate while others lagged. It’s a clear reminder of why teams must challenge the challenges faced by people in predictive analytics, especially when the world around them keeps changing.
Conclusion: Predictive Analytics Works, If You Avoid the Common Pitfalls
Predictive analytics has the potential to create serious business impact. But success doesn’t come from the algorithm alone. Most projects fall short because of issues like unclear goals, poor data quality, limited adoption, or disconnected systems. These aren’t just technical obstacles. They’re strategic challenges that need to be addressed early to avoid wasted time and resources.
At Kody Technolab, we help businesses turn predictive analytics into something practical and results-driven. Whether you’re starting fresh or trying to get an existing project back on track, we focus on solving the right problems, using the right data, and building solutions your teams can actually use. From planning to deployment, we ensure your predictive analytics efforts are aligned with your business goals.
If you’re looking to make smarter decisions and future-proof your operations, our team is ready to support you at every step.
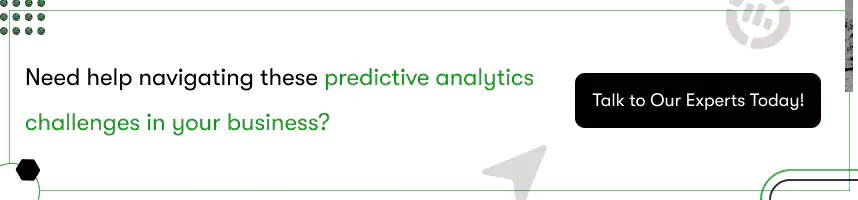